AI Arms Race Threatening the Machine Learning Ecosystem
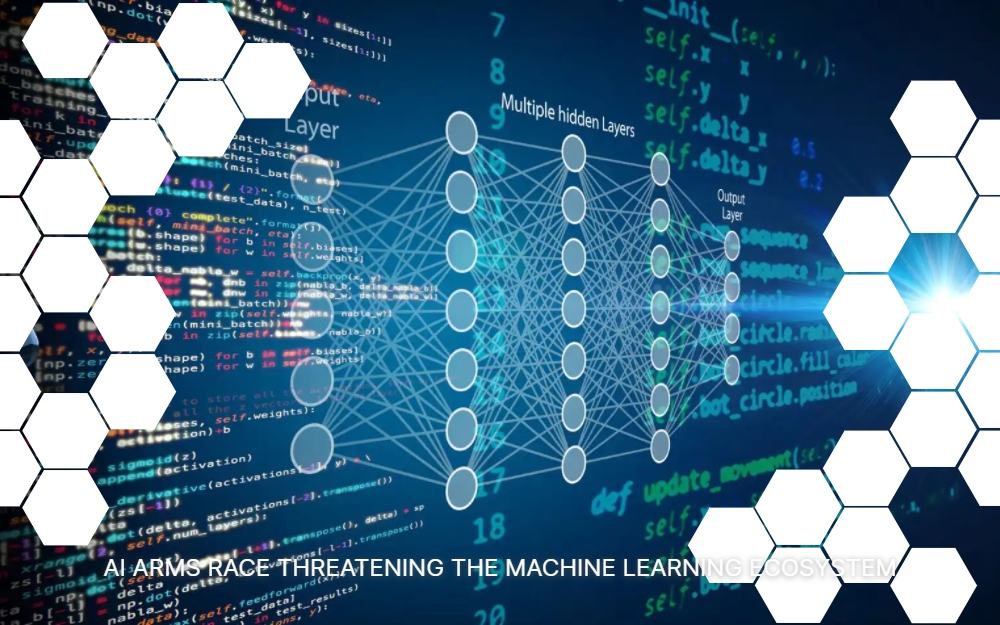
The ongoing battle between AI model developers, who extract knowledge from publicly available content, and content creators seeking to safeguard their intellectual property, poses a substantial risk to the stability of the current machine learning ecosystem. Experts are sounding the alarm about the potential consequences.
Defending Against Content Scraping and AI Training
In a recently published academic paper from the University of Chicago in August, computer scientists introduced innovative techniques to shield against wholesale content scraping, particularly artwork. Their goal is to thwart attempts to employ this scraped data for training AI models. The ultimate aim is to contaminate AI models that rely on such data, rendering them incapable of generating artwork in a similar style.
Data Pollution and Rising AI Adoption
Concurrently, another paper highlights the correlation between deliberate data pollution and the widespread adoption of AI, both by businesses and consumers. This surge in AI usage is transforming the landscape of online content, shifting it from predominantly human-generated to machine-generated. As AI models increasingly train on data generated by other AI systems, a recursive loop emerges, potentially leading to a catastrophic phenomenon known as “model collapse.” In this scenario, AI systems lose touch with reality.
The Deterioration of Data
The erosion of data quality is already in progress and could pose significant challenges for future AI applications, especially large language models (LLMs). These concerns have arisen in the midst of ongoing research into the issue of data poisoning, which, depending on the context, can serve as a defence against unauthorized content use, an attack on AI models, or a natural consequence of unregulated AI system utilization.
The Dual Nature of Data Poisoning and Style Cloaks
An illustration of the dual role of data poisoning emerges in a study focused on preventing the imitation of artistic styles without permission. Researchers at the University of Chicago devised “style cloaks,” an adversarial AI technique that alters artwork in a manner that causes AI models trained on such data to produce unexpected results. Their approach, named Glaze, has been transformed into a freely available application for both Windows and Mac, garnering over 740,000 downloads.
Balancing Act and Unintended Consequences
While the hope remains for AI companies and creator communities to strike a harmonious balance, the current trajectory of efforts may engender more challenges than resolutions. In much the same way that malicious actors can introduce deceptive or harmful data to compromise AI models, the widespread utilization of ‘perturbations’ or ‘style cloaks’ could yield unintended repercussions. These consequences span from the deterioration of the performance of valuable AI services to the emergence of complex legal and ethical dilemmas. The future of the AI landscape hangs in the balance as this struggle unfolds.
Check out our website New Facts World and follow us on Instagram for more exciting tech news.